Offering a Framework to Identify Investment Verticals and Uncover Opportunities in Generative AI
In the previous instalment of our “BPI Perspectives on Generative AI”, we navigated the Gen AI value chain in search for long-lasting products and defendable business from the viewpoint of BPI as an early growth investor. We established that we can expect further attractive VC cases to emerge on the Gen AI application layer: Essentially, startups at this part of the value chain should focus on creating unique, independent products that not only revolutionize existing user interfaces but also cater to underserved user groups. Moreover, building a defensibility flywheel through proprietary data acquisition and effective utilization, while integrating UI innovations in combination with user feedback loops is key for improved model and product performance. This strategy can especially bear fruit in domains where data ownership and generation are fragmented. However, in which industries or verticals can one expect such startups to emerge? In this BPI Perspectives piece, we aim to share some of our thinking around this question.
Gen AI models will impact many industries in various unforeseen ways. At this point in time, we don’t strive to create an exhaustive list of verticals to double down on, but rather outline some frameworks that help us navigate the coming wave of innovation.
When evaluating and prioritizing verticals for investing in Gen AI applications, there are certain fundamental factors to consider on a fundamental level:
- Market Dynamics: This encompasses both the current market size of the vertical and its projected growth. Obviously, industries with significant demand and anticipated expansion also offer attractive opportunities for the deployment of Gen AI applications.
- Current Technological Penetration: Industries that are already digitally mature or undergoing significant digital transformation are typically better positioned to integrate and leverage Gen AI powered technologies.
- Industry-Specific Challenges: An industry’s unique pain points, particularly in areas heavily reliant on processing of unstructured data or content creation, can inform the deployment of Gen AI. This technology’s potential to enhance and streamline these areas can add considerable value.
- Access to Proprietary Data: The extent to which an industry or vertical allows access to unique, valuable data sets is key. Startups capable of harnessing such data can secure competitive advantages, constructing defensible businesses via data network effects. This is especially attractive when data is located at various fragmented market participants.
- Regulatory Environment: The degree of regulatory freedom and flexibility plays a significant role, especially in industries such as healthcare or finance, where data usage and AI applications are or might become heavily regulated.
- Competitive Landscape: Understanding the competition and existing barriers to entry within an industry or vertical is crucial. Gen AI applications stand a greater chance of success in areas with limited competition (from tech companies!) or where existing solutions are outdated, particularly if they can offer superior performance, accuracy, or user experience.
- Monetization Potential: This relates to an industry’s openness to invest in and pay for generative AI applications, as well as the feasible revenue models that can be established. Industries valuing efficiency gains, enhanced customer experiences, or improved decision-making are more likely to offer viable monetization opportunities.
- Ethical Considerations: Finally, it is important to consider the ethical implications of deploying Gen AI across various industries. This includes potential misuse of AI, privacy concerns, risk of inherent bias, and issues of transparency in AI processes. Each industry will have unique ethical concerns, particularly those handling sensitive personal data.
A method we deploy to spot attractive verticals to build or invest in Gen AI powered applications is to think from the core capabilities of foundation models (view Figure 1). To that end, we begin with assessing the “Capability” of Gen AI foundation models (e.g. LLMs), essentially outlining the spectrum of outputs the technology can produce. This could range from text to image generation or even complex system simulations. The second step, “Function,” investigates the potential roles these AI-powered outputs can fulfill, whether it’s generating personalized content, automating design tasks, etc. The third step, “Vertical,” explores the various industries where the identified Gen AI capabilities and/or Gen AI-powered functions can add substantial value, such as in healthcare or entertainment. The final step, “Opportunity,” seeks to identify where the highest potential value lies for Gen AI applications, be it in the vertical-specific applications identified in the third step or cross-industry applications from the second step.
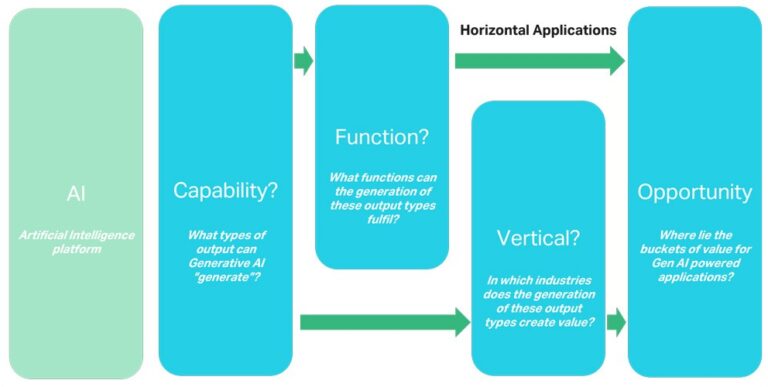
Figure 1 – Basic framework for navigating applications of Generative AI technology.
Capability:
Currently, foundation models, in particular transformer-trained large language models (LLMs), are particularly powerful when it comes to the generation of text-based content, including code (read more here). Therefore, as we prioritize Functions and Verticals in the medium term, our focus primarily lies on sectors that are more dependent on these forms of modalities, such as the legal industry (c.f. Figure 2). Nevertheless, foundation model for Image, Audio, Video, or 3D Asset Generation will unlock further lucrative use cases in the future.
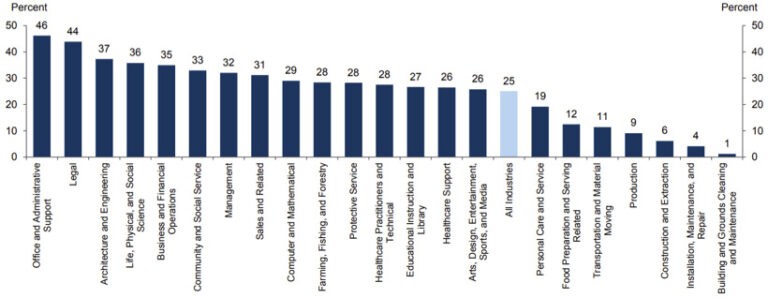
Figure 2 – Share of Industry Employment Exposed to Automation by LLMs (Goldman Sachs, March 2023).
Function:
When researching the landscape of VC-backed companies in the Generative AI space, you come across a plethora of market maps that do a fantastic job in providing an overview of startups across different AI-generated modalities (for example here, here, or here). Therefore, we will not dive too deep into this step of our analysis method and leave you with parts of our own segmentation in Figure 3 and Figure 4. It is noteworthy that most VCs and industry analysts portray the Gen AI subsegments along different functions fulfilled (e.g. Marketing & Sales Copywriting).
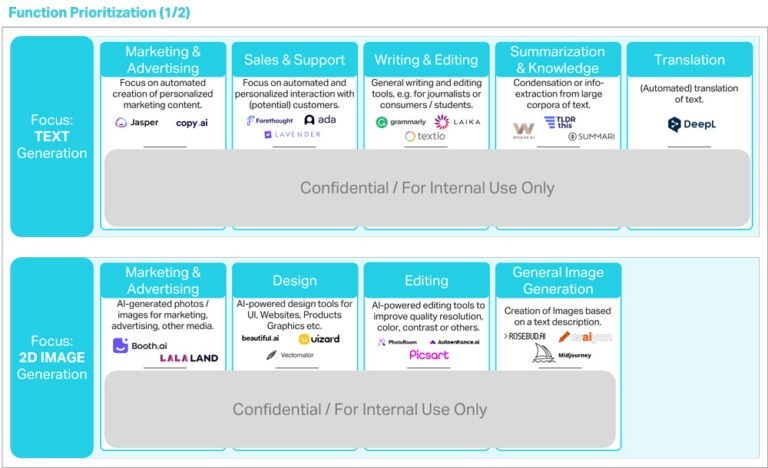
Figure 3 – Selection of Generative AI Functions enabled by AI-driven text and 2D image generation.
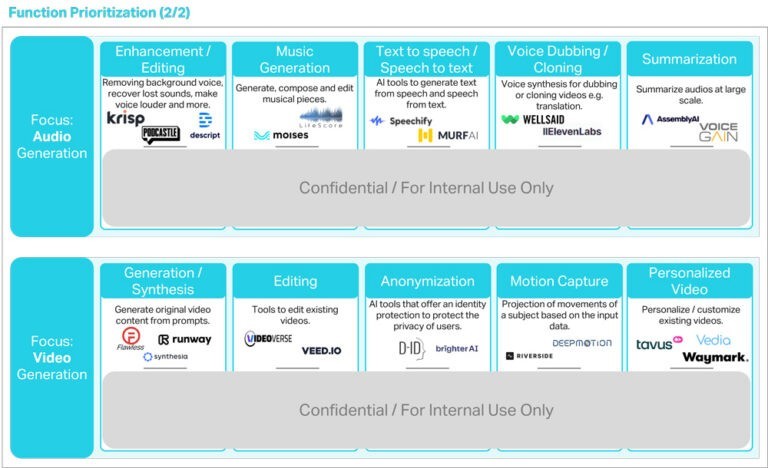
Figure 4 – Selection of Generative AI Functions enabled by AI-driven audio and video generation.
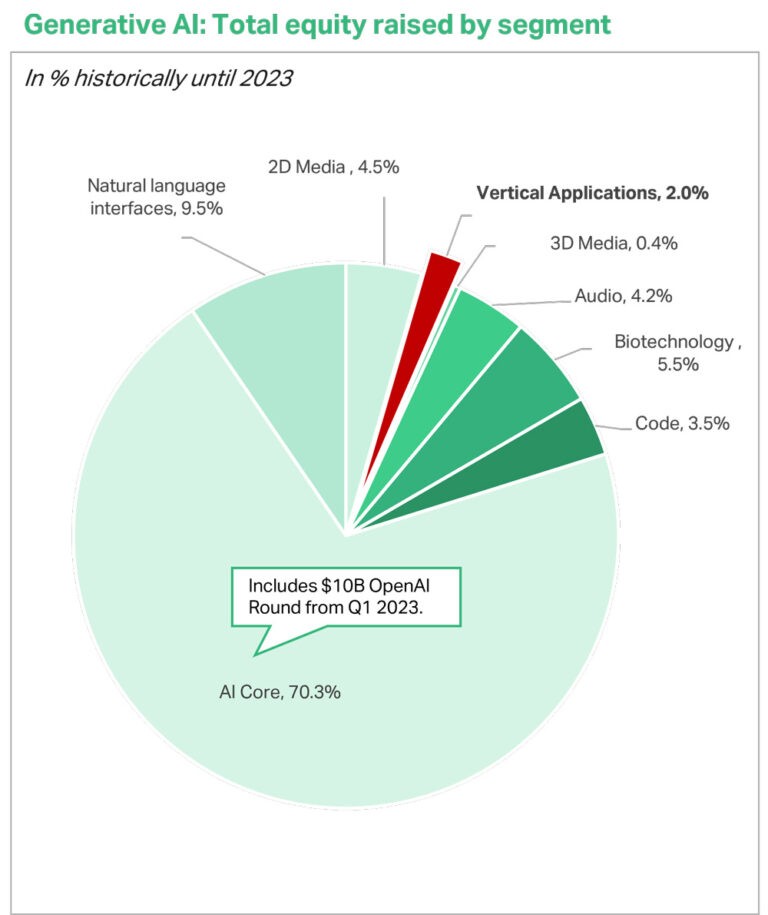
Figure 5 – VC funding by Generative AI Segment (Source: Pitchbook, April 2023)
Also, when it comes to capital being deployed in the space (c.f. Figure 5), most funding allocated on the application layer is put to work in horizontal use cases tackling various industries with function-specific generative capabilities (e.g. customer support or sales communication). In some of the more obvious segments we already identify a very high number of early-stage startups (e.g. up to 140 for text generation for marketing purposes). This should be kept in mind when evaluating the competitive landscape factor mentioned above.
Vertical:
So far, most VC-backed companies within the Gen AI landscape are either active at the core infrastructure layer or providing horizontal applications across industries (c.f. Figure 5). However, we expect more generative AI applications to emerge for domain-specific use cases as the core infrastructure platform matures.
Two reasons for this belief go back to the core evaluation-factors outlined above: (1) Tackling of industry-specific challenges and (2) Access to unique proprietary data. Vertical-specific products will be better equipped to leverage domain-specific datasets to create or finetune more precise and defendable models. Additionally, companies focused on specific verticals or domains may be more able to come up with novel and unique user interfaces between customers and models to solve industry-specific challenges. These user-interfaces don’t need to be as flexibly applicable as those for horizontal use cases catering to multiple industries, further raising barriers of entry. However, the addressable market size of vertical applications is likely to be scrutinized, due to their narrower focus.
So far, we identified 10 verticals where we already see exciting domain-specific Gen AI applications emerging (view Figure 6 and Figure 7). While we are interested in both horizontal and vertical applications across domains, BPI will keep with its core investment hypotheses and focus on companies targeting consumers or businesses via product-led customer acquisition. We are looking for solutions that have a clear monetization potential and products that can potentially be internationalized. When considering the verticals outlined in this context, BPI has already successful invested in FinTech (e.g. Moneybox), Cybersecurity (e.g. Nord Security), EdTech (e.g. Skillshare), PropTech (e.g. Habyt), Fashion (e.g. Vinted) and are highly interested in Gaming and LegalTech (read more here).
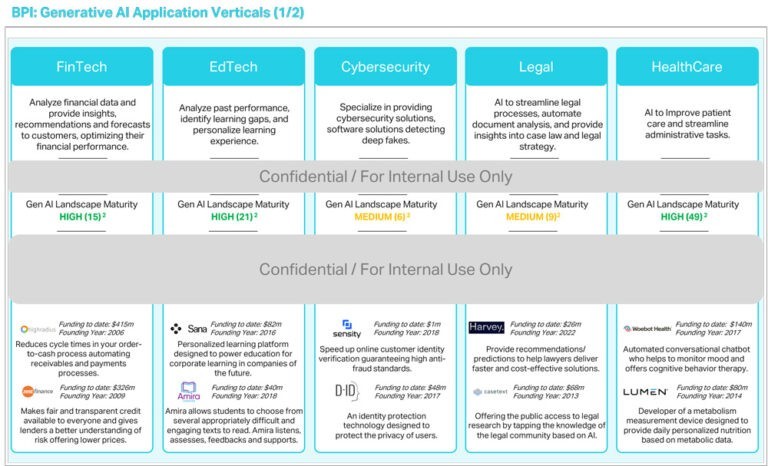
Figure 6 – Generative AI Verticals (1/2) | Number of identified startups.
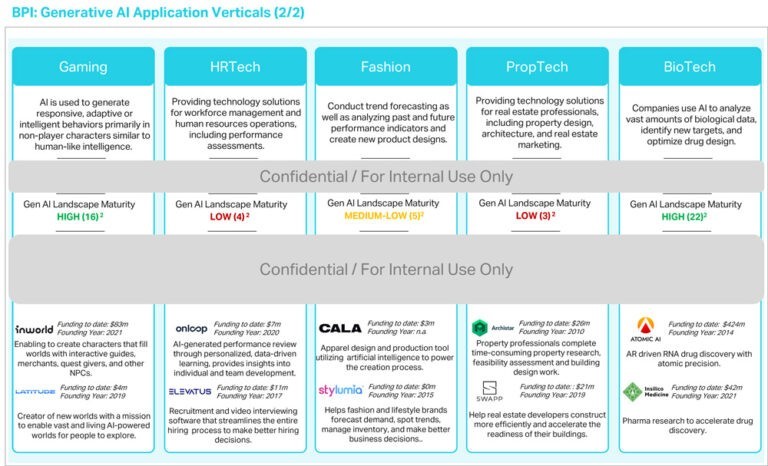
Figure 7 – Generative AI Verticals (2/2) | Number of identified startups.
As we continue to explore this landscape, we are eager to connect with startups and investors who share our excitement about the promise and potential of Gen AI. Please reach out to us at investments@burda.com. We look forward to being part of this journey with you.