Unleashing the power of Generative AI: Navigating the value chain for long-lasting products and defendable businesses.
As you have seen from our previous BPI Perspectives instalments, we – like most other VCs – have been following the Generative AI wave for some time now. From the start, the two debates we most commonly have when evaluating start-up investments within the Generative AI space are: “Is this a feature or a long-lasting product?” and “Can this product become a defendable business that matters?” To think about these questions when analysing growth investments in Generative AI, we can use the value chain depicted in Figure 1 as a mental guideline.
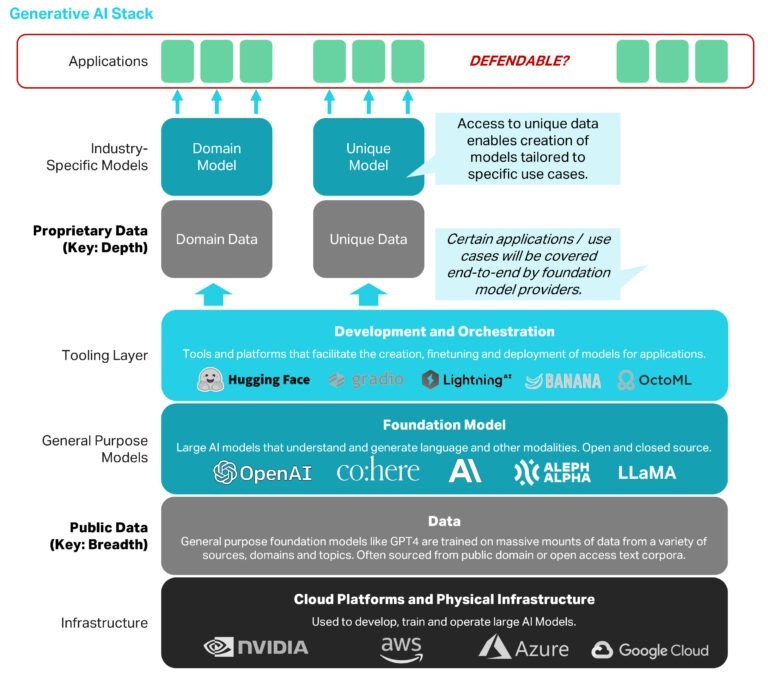
Figure 1 – Generative AI Value Chain. Proprietary data is one key to defensibility on the application layer.
“Is this a feature or a long-lasting product?”
Foundation models, most commonly large language models (LLMs), are general purpose technologies that enable the integration of AI capabilities into products with limited effort and without the need for scarce ML talent. Open-source models (e.g. LLaMA) are catching up more and more with their closed-source competitors, further increasing the accessibility of Generative AI capabilities and lowering barriers of entry to the space.
On the application layer, this has led to the emergence of many new Generative AI start-ups. This wave is already creating red ocean markets, for example in the customer support space. We see two additional trends that one should keep in mind in this context:
First, incumbents have been quick to sign partnerships with OpenAI, Anthropic & Co. to add Generative AI features to their existing products. Examples include Adobe, Salesforce, Notion, Microsoft and many others. Incumbents – especially big tech companies – benefit from strong digital distribution and large datasets that can be leveraged to finetune models for the respective use cases. These advantages can be reaped especially, if Generative AI capabilities can add value within the incumbents’ existing user interfaces. The speed at which these new features were added also speaks to the amount of heavy (ML-) lifting that is already covered at the foundation model layer.
“Every incumbent tries to make the new thing (Generative AI) a feature of the old thing, and every incumbent has read the Clayton Christensen ‘Disruption’ book and wants to make sure they make the jump” – Benedict Evans (May 2023).
Second, foundation model providers, such as OpenAI, are not only providing a fundamental technology for other companies to build applications on top of. They are striving to become end-user platforms themselves. OpenAI’s Chat GPT is a well-known example for this trend. The tool – originally meant to be a research demo to showcase the capabilities of OpenAI’s LLMs – directly provides many features and capabilities that are also offered by stand-alone apps, e.g. writing tools. The introduction of plug-ins (e.g. of OpenTable or Instacart) to ChatGPT is another step towards the establishment of a new consumer platform (read more of our thoughts on this here).
Despite these two trends, we believe in the creation of new independent products in the Generative AI space. Incumbents have less of an advantage if the capabilities of Generative AI can only be leveraged to solve a customer problem by radically changing the exiting user interface (Heartcore discusses this deeper here). Additionally, new products may employ Generative AI capabilities through new UIs to address user groups that were previously completely underserved by incumbents.
Additionally, the tooling-layer that enables incumbent and new entrants to tap into the opportunities of generative AI (e.g. by facilitating the finetuning of foundation models based on proprietary data) represents a new investment opportunity for SaaS VCs. So far, most of VC investment in Generative AI has been allocated to the – still maturing – tooling and platform layer. According to Pitchbook, >$4b of venture capital (not counting >$10b for OpenAI) flowing into Generative AI to date have been invested in tools and platforms for data management, infrastructure, and end-to-end platforms for model development, deployment, and management. Vertical applications received only 10% of that so far, suggesting that we are still in the phase of building the new platform.
Even if we see the opportunity for independent products on the application layer, the question remains whether these products can also be a valuable business.
“Can this product become a defendable business that matters?”
Once we have established that the solution provided by a start-up is not better suited to be a feature of an existing company, we need to understand whether this product has the potential to create long-term economic value. The primary criterion to do so is to decide whether the company can create a moat around its business.
This question is probably the most talked about by VCs looking at the Generative AI wave but also at AI in general (e.g. by VCs from La Famiglia, PointNine and Cavalry here, here, here, and here).
What most investors agree on is that defensibility boils down to two key factors: having exclusive access to valuable proprietary data and effectively utilizing that data to create a superior product. Start-ups can establish a defensibility flywheel by generating more data through increased product usage and leveraging that data to enhance the appeal of the product, thereby further boosting its usage. Additionally, incorporating user interface (UI) innovations that incorporate human feedback loops (RLHF) can kickstart this defensibility flywheel, leading to improved model performance and, consequently, product performance.
Start-ups striving to create a defendable long-term business need to find ways to aggregate unique and proprietary datasets that they can use to fine-tune foundation models to create superior customer experiences. Start-ups targeting specific domains or industries – especially ones where individual incumbents and potential customers have limited or siloed datasets – may be especially successful in that regard by generating data network effects across many fragmented datasets.
As apparent from our portfolio, we have always been focused on investing in companies that ‘own’ the end-user relationship (across B2C, B2B2C and B2B/B2SME businesses) and build vertical category leaders. In the context of what we discussed above, we see a strong advantage in being consistent with our strategy and look forward to the wave of innovation coming our way in the Generative AI Space.
We are especially excited about defendable vertical applications (we will discuss the different verticals in Part IV) and product-led SaaS tools that enable existing companies to make the most of this newly emerging.
If you are a founder or investor in the space and want to connect with us, please reach out at investments@burda.com.